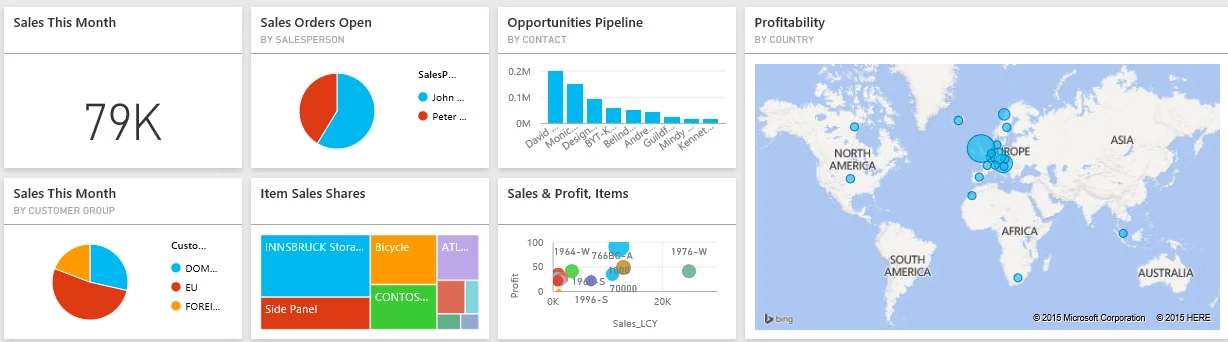
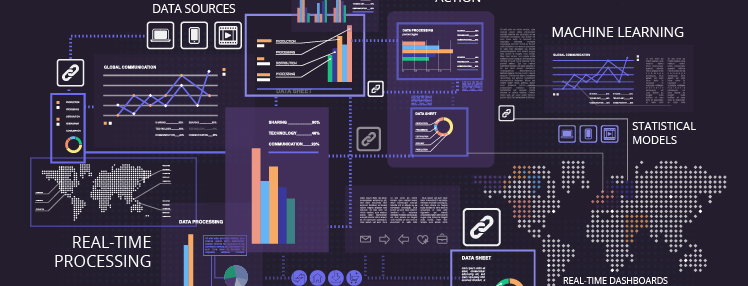
Data Science is not a goal, but a tool
Building data science teams may be a daunting task – both because of analytics’ transformative power and our species’ tricky fear of change.
Yet data science is not a goal: it is merely a tool to achieve better business outcomes. This especially true – and especially important to grasp – for non-technical companies trying their hand at data-driven decision making.
I couldn’t think of a better example than the recent talk by Brian Hovis, VP of Marketing at Nordstrom (a US chain of department stores since 1901, 2017 sales: $15.1B).
“We have invested in a data scientist capability in-house. That doesn’t happen overnight. You have to source that talent, onboard that talent, and build that capability”. As said by Hovis, about the company’s venture in exploring 100 years’ worth of customer data.
But Nordstrom, given they are fully capable to custom-build machine learning (ML) and artificial intelligence (AI) tools, decided to go for a different approach. The dedicated team of data scientists were directed to use existing third-party products instead of venturing into the wild with a non-defined solution to a somewhat ambiguous task like “increase sales”.
“We would recognize that we are a retailer, we’re not a technology company,” Hovis shared with the audience at Transform 2018, also stating that certain investments in things like building AI software from the ground up wouldn’t make sense financially. Nordstrom needed to keep focus “without spinning [its] wheels”.
THE LEAN WAY
This approach makes a lot of sense for businesses around the globe – loosely dubbed “lean” by entrepreneurs and CEOs alike, the goal is to prove business value as early as possible – with the least amount of investment. A 100-year large database is ripe for analytics: but would insights bring real, hard income?
Certain companies choose to take an even leaner approach – have their proof-of-concepts developed hand-in-hand with an external partner, validate findings, improve initial results – and only then internalize the process. This is a risky proposition, taking into account that industry-specific knowledge is critical when building a world-realistic model. So organizations need to choose their data science partners wisely – and also define specific business cases.
Another key prerequisite is the ability to trust your partner with your company’s most confidential data – including financial and customer information. Most of the insights we were able to produce for our customers came through data enrichment and cross-source analytics – for example, an optimization of the sales force for undervalued existing customers in one case.
NORDSTROM: SMALL STEPS, BUT STEADY
In Nordstrom’s case, the first step was to segment the huge customer base and identify customers that were more likely to shop from the outlet brand – “Nordstrom Rack”. While not all customers would be looking to pay the full price of a product, they may still sufficiently appreciate an item to look for discounts for it. That’s where remarketing efforts of the outlet brand comes in – via email or phone, depending on customer preference. In retail, acquiring a customer is quite expensive, so companies are looking into nourishing their existing ones, even if it means diminished margins.
The results in this case? While no numbers are shared, actions speak louder than words: Nordstrom is expanding AI application across its business, including in fulfilment, distribution, and customer experience.