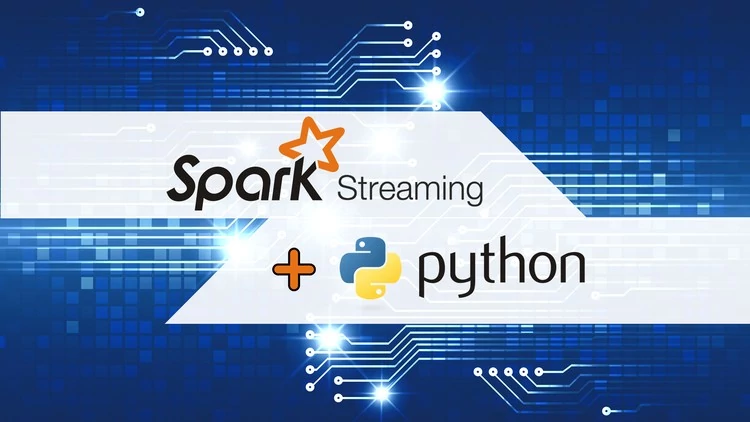
Scraping data to marketing insight
Data comes in all shapes and forms these days: machine-readable or not, non-relational or structured, document-based or even analog. With the abundance of data companies need to cautiously assess what data would bring added value. For example, some might improve their operations and strategy – while some might constitute a waste of storage, resources and product development effort.
At Code Runners, our mission is to facilitate data-driven decision making. We do it by collecting, transforming, securing and visualizing datasets of varying origin and complexity. When it comes to marketing and market research – industries we proudly serve – some very valuable data is readily available online. A good example here are product reviews by users around the globe. The only restriction? The researching party needs to scrape and ETL the data before analyzing it. As a final step, it comes up with improvement suggestions based on customer feedback.
One such project involved collecting data from the top 3 e-commerce platforms in fifty countries – a total of 150 sources! – and devising automated triggers that monitor customer feedback to enable early detection of performance dips. Following a lean methodology, we decided for static triggers. However, that decision also meant we needed to transform and compare extracted data in a scalable way – and run analysis on top of it.
When building crawlers, there are a few considerations to make:
Read the T&C
Scraping is not illegal, but it may be against the terms & conditions of certain e-commerce sites. While some provide APIs and monetize the data they have, that’s not always the case. Smaller platforms try to limit crawling (throttling, IP banning, etc.) simply because they can’t handle the extra traffic (it’s estimated that 60% of traffic online comes from bots). In any case, the first step should be to ensure legal compliance.
Collect data first, analyze it afterwards
From a process perspective, the application should split crawling into fetching web pages and analysis. There are multiple reasons for that, the main one being future extensions to the dataset (e.g. building a user graph or analyzing review sentiment). While it might be possible to re-scrape the content from scratch, it will be difficult to retrofit the whole environment unless it has been actually stored.
Another great reason is scalability – once the crawler goes live, the two phases would require different execution times and resource allocations. Microservice-based or even serverless architecture may provide sufficient flexibility to tailor processor, memory and disk I/O allocation. With a monolith application, both resource requirements and operational cost will spike.
The last reason I’ll highlight is extendability: splitting things early allows agility and extendability as needed. It is likely that the scraping module will run around the clock and would require heavy parallelization; it should be able to scrape multiple sources and use a pool of proxies; update roll outs and backwards compatibility should be possible without unnecessary downtime.
Avoid in-memory persistence
Keeping too much data in memory, clogs performance, spikes resource usage and runs the risk of losing data if the process fails. We recommend using fast-access key stores (think Redis), but on-disk persistence within a database is also a viable option. For microservice-based architecture, RabbitMQ may be a situational alternative. If the amount of data collected is extremely large, Delta Lake should be considered: developed by the authors of Apache Spark. As one might expect, both technologies get together well.
Once a page is crawled, it should find its way to the disk I/O as soon as possible – document-based databases are the optimal choice here. After the HTML extractors complete, the data is good to go to Spark for deeper analysis.
The same goes for logging – files are better than STDOUT, because you can control , analyze and archive them in a structured manner.
Catch and handle exceptions gracefully
When operating at high velocities and threshold resources for days, it is critical to monitor performance and ensure you’re not wasting time and resource in vain. The ability to notice errors as soon as they happen is pivotal in ensuring the project’s long-term success and actual quality of insight. Early alerts may include HTTP error responses, malformed HTML or changes in the source structure.
Start small, scale and adapt as you go
If the goal is to crawl 10’000’000 products daily, one shouldn’t start with the whole set right off the bat – it’s much better to start small and handle issues as they come. Analyzing bottlenecks in-depth is key: more often than not, changes will push the application against system limitations: CPU, memory, disk IO or network IO.
Putting it all together
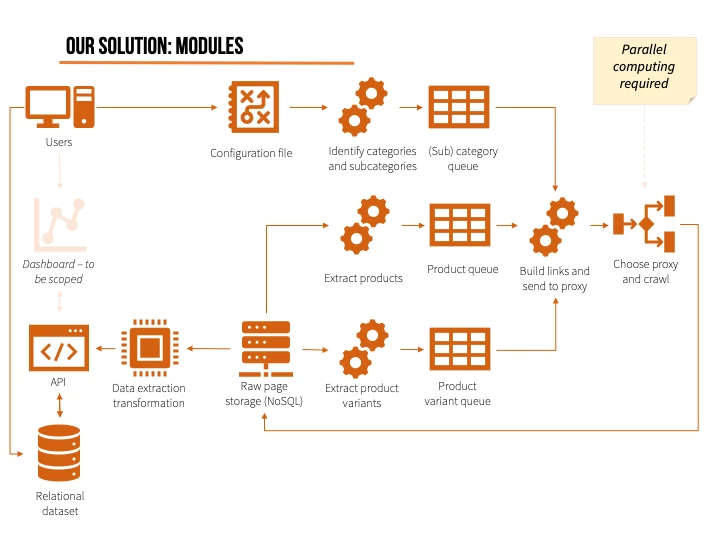
With all considerations in mind, here’s the architecture for a tool that collects competing products across categories. Details like cookie management, technology or platform choices are omitted on purpose, but you can always ask us for more details!